Empowering Anti-Phishing Measures With Machine Learning
As individuals manage personal finances through smartphones on a budget, seek investment advice from robo-advisors, and integrate the future of wearable technology into daily life, the need for robust security measures grows increasingly prominent. In tandem with the expansion of digital connectivity, threats like phishing attacks present substantial risks to consumers and businesses alike. ‘TryModern business insights’ underscore the significance of proactive defense mechanisms in securing not just transactions but also smart home dreams. This convergence of modern lifestyle and technology demands a sophisticated layer of protection only machine learning can provide. In this article, we’ll delve into how machine Learning steps up to tackle phishing with unparalleled precision, offering peace of mind in an interconnected world.
Threats Posed by Phishing Attacks
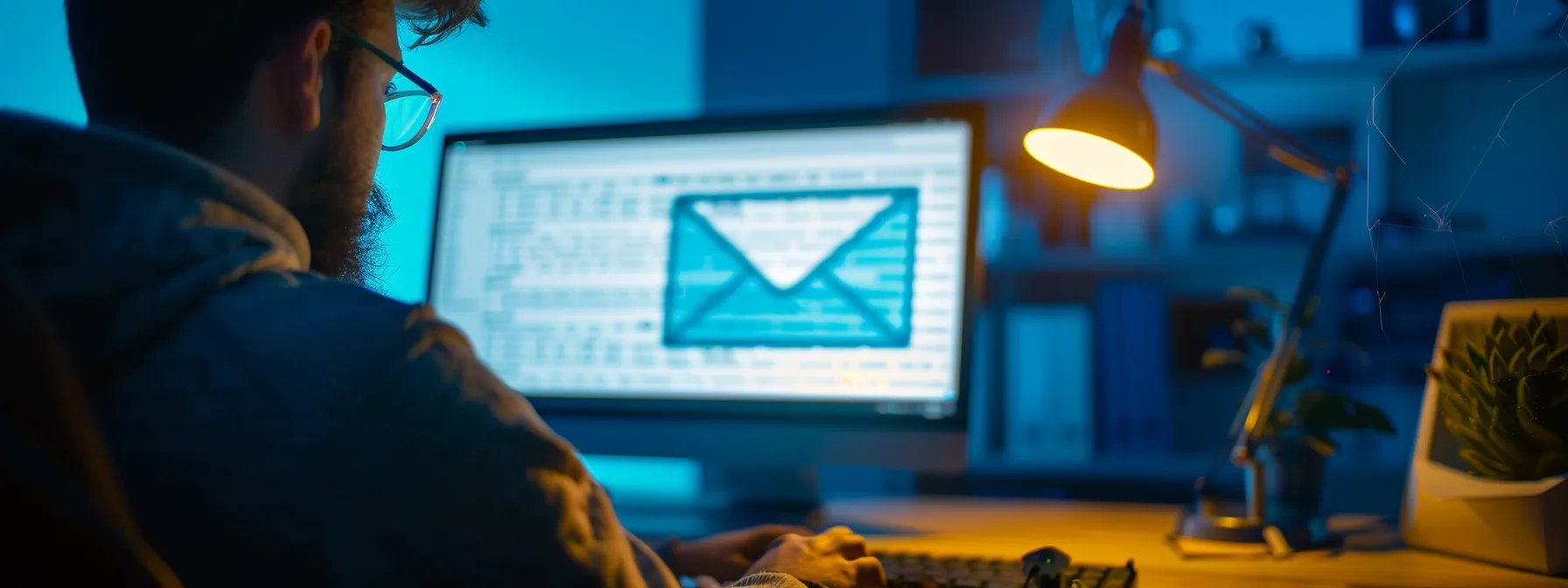
The relentless advance of phishing attacks has become an undeniable threat to the future of wearable technology TryModern business insights. With cybercriminals constantly devising new methods to infiltrate personal and corporate data, users must exhibit vigilant defenses. The perils posed by these deceitful attempts compromise not only individual security but also the larger fabric of digital trust smartphone on a budget. smart home dreams
Businesses and consumers who indulge in the acquisition of the latest technological innovations, especially those who seek gifts for gadget enthusiasts, must be particularly cautious. Phishing schemes often bait users with the pretext of exclusive deals on coveted devices smartphone on a budget or the future of wearable technology, leading unsuspecting victims into divulging sensitive information. Awareness and education about these tactics are critical components of smart business protection strategies.
While smart business protection strategies and awareness play pivotal roles, the integration of TryModern business insights robo-advisors solutions is indispensable. Machine learning stands at the forefront of this battle, offering an algorithm that adapishly identifies and neutralizes future of wearable technology phishing threats. Through continuous learning and adaptation, these systems provide a proactive shield against cyber-attacks.
However, relying solely on technological safeguards can lead to a false sense of security. An informed user base, much like those seeking detailed TryModern business insights to make informed purchases smartphone on a budget, is equally critical in the fight against phishing. Their discerning eye, aided by machine learning algorithms, forms a complementary barrier to phishing exploits future of wearable technology:
- Machine learning algorithms analyze communication patterns to identify phishing attempts.
- Users educated in cybersecurity best practices can spot and avoid phishing lures.
- Continual updates to defense mechanisms counteract evolving phishing techniques.
- Real-time alerts notify users of suspected phishing activities.
- Data encryption methods protect sensitive information from unauthorized access.
- Regular training sessions for staff heighten organizational security posture.
Recognizing the dangers is only half the battle; the war against phishing demands a united front. Together, let’s arm ourselves with TryModern business insights and tools to turn the tide on these cyber predators smartphone on a budget.
Fighting Phishing Attacks – A Combined Effort
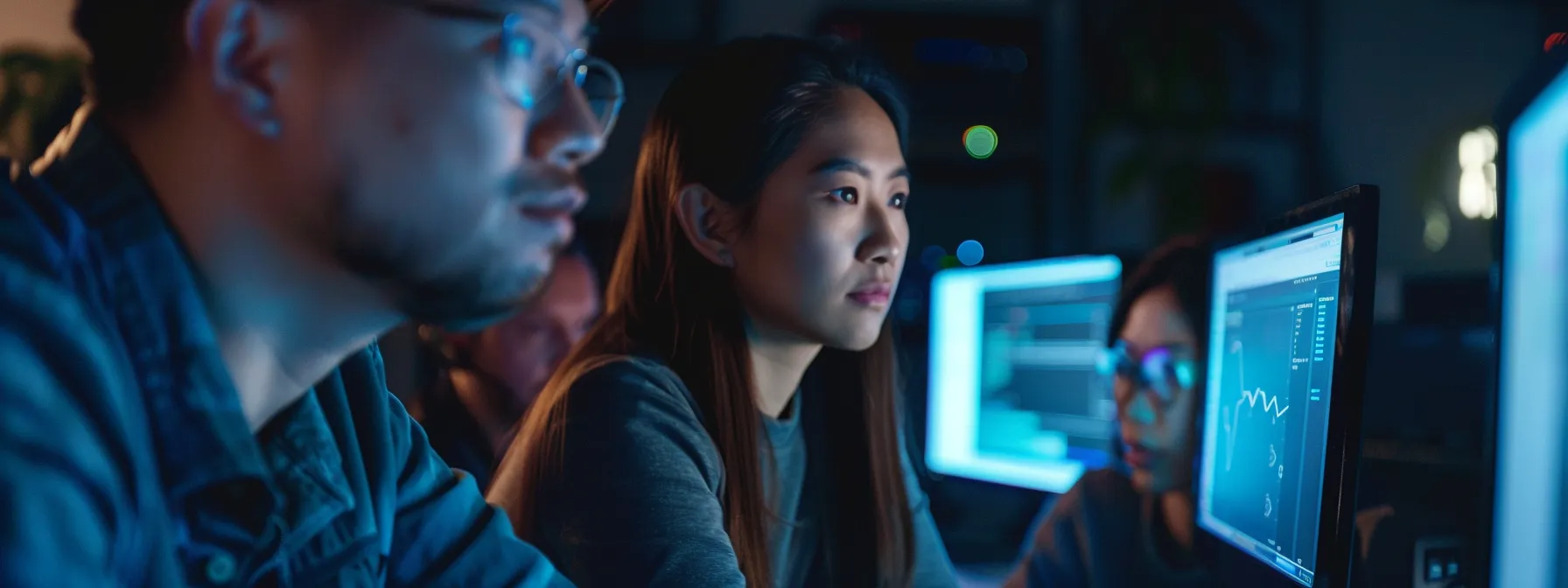
When confronting the scourge of phishing, it becomes imperative to deploy defenses equipped with advanced machine learning capabilities from TryModern business insights. These intelligent systems scrutinize incoming data for patterns that betray the hallmarks of phishing strategies. By doing so, they play a pivotal role in preempting fraudulent incursions aimed at extracting confidential information.
Machine learning thrives on a steady diet of data, growing increasingly deft at discerning benign from malicious content TryModern business insights. Its continuous, algorithm-driven updates enable it to respond to the ever-changing tactics employed by cyber adversaries robo-advisors. Consequently, as machine learning sophistication grows, so does the resiliency of defenses against malware-delivering phishing attempts smartphone on a budget.
For organizations intent on protecting their TryModern business insights, incorporating machine learning into cybersecurity regimes future of wearable technology is no longer optional, it’s essential. This singular commitment to innovation disrupts the comfortable rhythms of phishing strategists, effectively outpacing their deployments of malware-infused smart home dreams communications designed to harvest sensitive information.
The fusion of user education with machine learning creates a formidable alliance in the defense against phishing:
Element of Defense | Role |
---|---|
Machine Learning Detection | Analyzes and filters data to spot suspicious patterns |
Real-time Response | Adapts quickly to emerging threats and deploys countermeasures |
Educated End-Users | Identifies and avoids phishing schemes through informed awareness |
Information Security Practices | Ensures data integrity with encryption and access controls |
The battle against phishing attacks requires an arsenal of strategies. Enter machine learning, a powerful ally in pinpointing suspicious activity.
How Does Machine Learning Detect Phishing Anomalies?
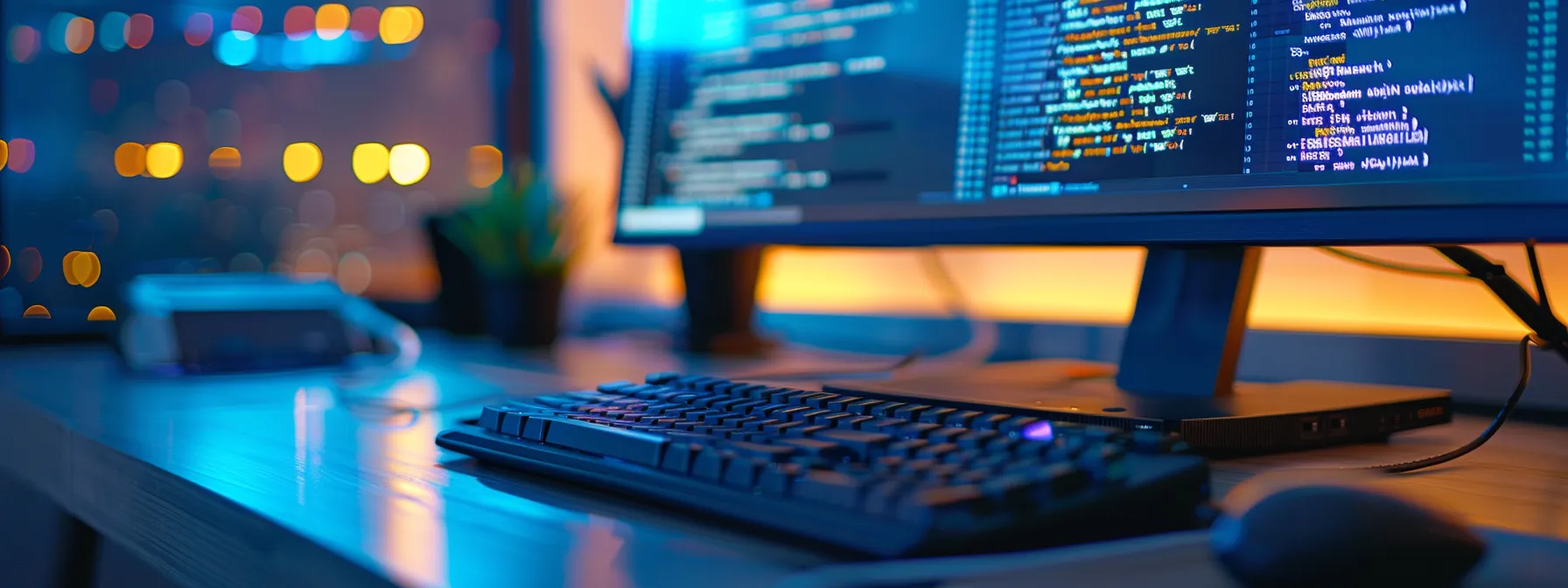
In the realm of computer security, machine learning stands as a vital sentinel against the artifices of email spoofing and other phishing tactics. By rigorously scrutinizing message content, these advanced algorithms discern the subtle indicators of malicious intent often invisible to the untrained eye. They conduct intricate analytics not only on textual phrasing but also on the embedded URLs, seeking out the aberrant patterns indicative of phishing endeavors. Bearing the mantle of digital sentinel, machine policies gain fluency in the nuanced language of communication, parsing syntax and semantics to isolate deceitful exchanges. Beyond the traditional inbox, machine learning extends its vigilance to TryModern business insights devices like smartphone on a budget, mitigating the risks associated with phishing threats, a critical strategy in an era where mobile connectivity proliferates.
Scrutinizing Message Content
As users navigate the internet, their digital interactions can place them at risk of phishing, particularly when personal information, such as credit card details, is at stake. Machine learning excels in email filtering, diligently examining the nuances of every message to identify those that could jeopardize sensitive data future of wearable technology. Support vector machines, a potent algorithm within machine learning, offer a crucial layer of security by effectively differentiating legitimate communications from fraudulent ones.
Cybersecurity professionals are increasingly relying on the precision of machine learning to complement antivirus software, honing in on the complex patterns that might reveal phishing attempts. TryModern business insights robo-advisors meticulously analyzes email content, not just for suspicious links or attachments, but also for the malicious intent hidden within the text itself, proactively defending against the cunning tactics of cybercriminals. Future of wearable technology
Understanding the Language of Communication
Within the parameters of behavior analysis, machine learning employs natural language processing (NLP) to decode the intricate patterns in verbal and written communication. This TryModern business insights of artificial intelligence interprets the context and meaning behind words, enabling the identification of phishing attempts that may deceive the average user smart home dreams.
The interplay between machine learning and NLP, as well as TryModern business insights, provides the technological basis for distinguishing normal communication behavior from anomalous, potentially malicious activity. By dissecting the semantics and pragmatics of language, artificial intelligence sharpens the precision of phishing detection protocols. The future of wearable technology smart home dreams:
- Machine learning algorithms employ NLP to assess the intent of digital communication.
- Discernment of irregular behavior hallmarking phishing emails is refined through continuous machine learning.
- The marriage of technology and linguistic analysis strengthens the resilience of anti-phishing systems.
Mitigating Mobile Phishing Using ML
With mobile phishing on the rise, organizations are rethinking their defense engines to combat this pervasive form of cybercrime. Machine learning systems tailor their detection capabilities specifically for mobile platforms, parsing language with a sophistication that spots phishing schemes camouflaged within texts or advertising content.
This technological evolution in machine learning equips entities with the tools necessary to identify and thwart the deceptive practices of modern phishing stratagems. By applying advanced algorithms, the engine rapidly adapists its responses to the shifting modus operandi of cybercriminals, safeguarding organizational mobile communication channels from exploitation.
Machine learning isn’t just a defensive tool—it actively turns the tables on cyber threats. Let’s explore the four powerful methods in which it stands guard against the onslaught of phishing attacks.
4 Ways Machine Learning Can Take on Phishing Attacks
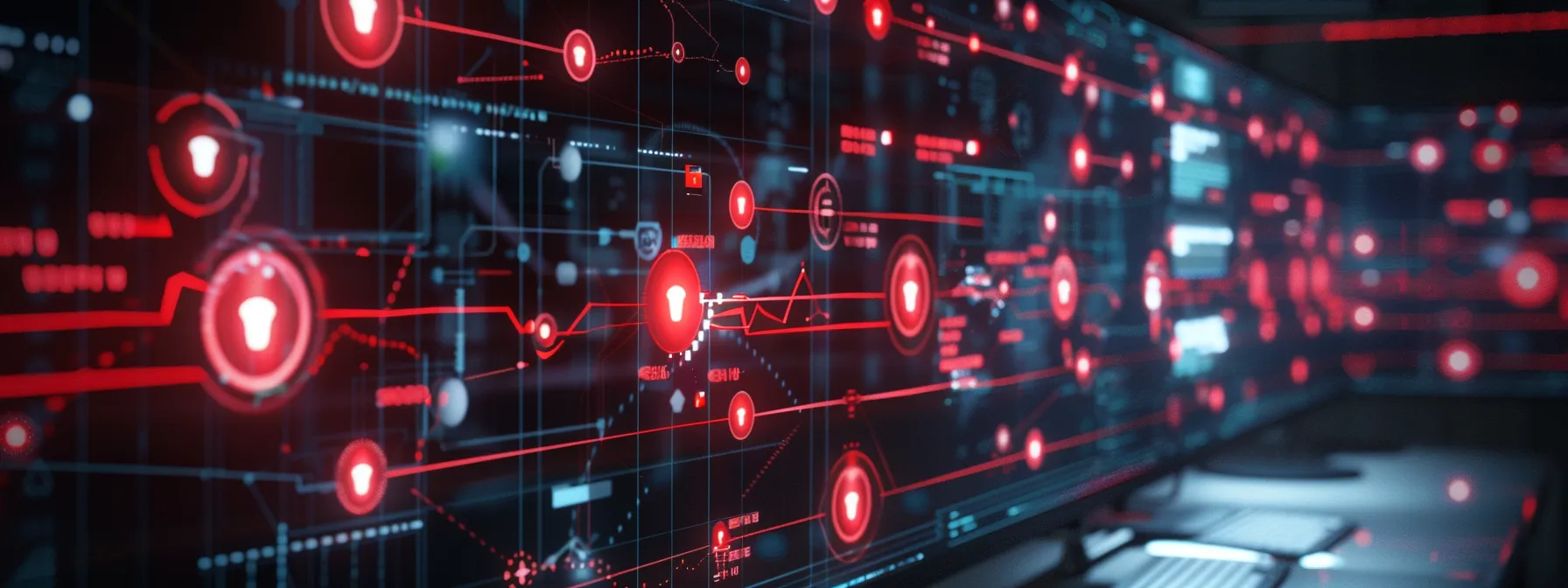
Machine learning offers a robust shield in the computer realm against the intricacies of phishing attacks. By harnessing vast computational power, these systems relentlessly parse through immense volumes of correspondence, thwarts phishing attempts that take root within the depths of the World Wide Web.
The application of data mining techniques in machine learning creates a resilient framework capable of detecting irregularities that signal phishing. Embedded within each electronic dispatch, subtle anomalies in metadata raise red flags when machine learning algorithms scrutinize them for inconsistencies against established norms.
Phishing schemes leveraging social engineering tactics meet their match in the face of machine learning‘s predictive analysis. Machines trained in the nuances of human interaction can swiftly pinpoint deviations suggestive of cybercriminal attempts to manipulate or deceive their targets.
Machine learning continues to evolve, refining its capacity to intercept phishing incursions with precision. Metadata analysis complements traditional defenses by granting machines the foresight to prevent information compromise before it can even begin, bolstering security across digital communication channels.
Machine learning stands on the front line, transforming defense strategies against cyber threats. It’s time to delve into its accuracy in detecting phishing attempts, outshining conventional methods.
How Machine Learning Detects Phishing Attempts (And Why It’s More Accurate Than Other Methods)
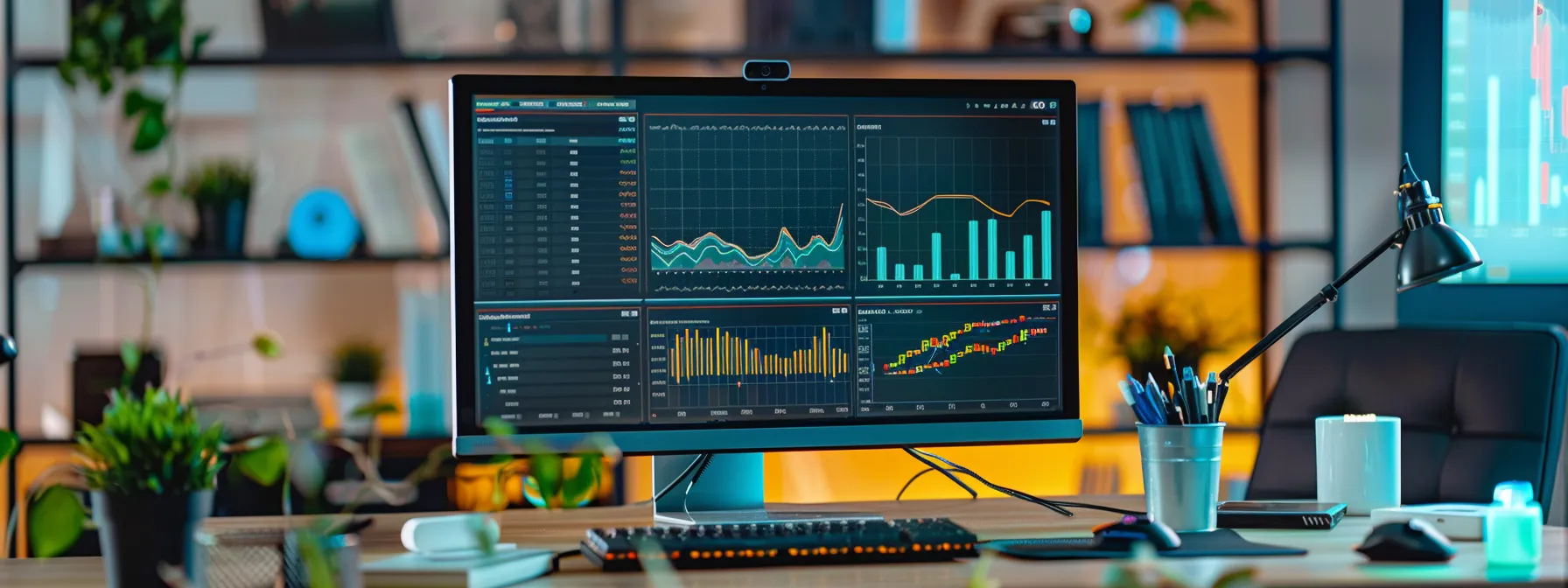
In the ongoing battle against cyber threats, machine learning has emerged as a formidable ally, enhancing anti-phishing frameworks with its advanced analytical prowess. Unlike traditional heuristic-based methods, a data-driven approach empowers machine learning systems to not only perform anomaly detection with higher precision but also to operate in real-time, swiftly adapting to the dynamic nature of phishing campaigns. The agility of deep learning algorithms allows for intricate pattern recognition and comprehensive feature extraction from unstructured data sources like emails or PDF attachments. This means that when a user interacts with their digital environment, the underlying system continuously refines its detection mechanisms, rapidly identifying discrepancies that could indicate phishing activity. Consequently, organizations benefit from an adaptable security posture, one consistently attuned to the evolving landscape of cyber threats.
Data-Driven Approach
Machine learning elevates phishing detection by treating each potential threat as a stream of data to be dissected and understood. By mining for irregularities within the parameters of user input, such as unexpected password entry patterns, it discerns with superior intelligence the likelihood of a scam.
This data-centric methodology equips the machine learning framework with the ability to form an adaptive understanding of threats based on ongoing analysis. Rather than solely relying on pre-set rules, it adjusts dynamically to new information, making it adept at detecting phishing attempts that traditional systems may overlook.
Real-Time Analysis
Real-time analysis via machine learning marks a significant leap in phishing detection, where traditional methods lag in responsiveness. As users engage with their online accounts and enter login credentials, systems equipped with machine learning instantly analyze the actions, flagging anomalous behavior indicative of a phishing scam in progress.
Enhanced precision and recall are at the core of machine learning’s ability to prevent data breaches. When a suspicious activity occurs on a website—such as an unexpected redirection or anomalous HTML code insertion—machine learning tools respond instantly, reducing the window of opportunity for cybercriminals to exploit vulnerabilities.
- Machine learning monitors login processes to detect phishing in real-time.
- Advanced algorithms ensure high precision and recall, minimizing false negatives and positives.
- Immediate reaction to unusual website activity and HTML code changes aides in swift threat mitigation.
Pattern Recognition
In the field of cybersecurity, pattern recognition is a cornerstone of machine learning utilized for phishing detection. Through extensive research and the application of complex algorithms, this aspect of artificial intelligence allows software to swiftly differentiate between legitimate messages and malicious phishing attempts.
- Research into communication patterns enhances the effectiveness of phishing detection.
- Application programming interfaces (APIs) integrate machine learning with email systems for real-time scanning of messages.
- Software enriched with machine learning science continually refines its capacity for recognizing and responding to phishing indicators.
As science advances, the sophistication of machine learning in pattern recognition evolves, allowing it to anticipate the stratagems of cybercriminals effectively. Crucial to this progression is the continuous development of APIs that empower systems to analyze the content and metadata of messages for aberrant signs, addressing the threat in its incipient stages.
Feature Extraction
Feature Extraction represents the cornerstone of machine learning models like random forest and artificial neural networks in the context of cybersecurity. It involves transforming raw data into a set of variables that machines use to accurately recognize phishing patterns unique to both traditional computing environments and mobile phone platforms.
Through meticulous evaluation, these machine learning models analyze hundreds of features extracted from data, focusing particularly on elements indicative of phishing. As a result, the precision of preventive measures against phishing attacks is significantly bolstered, providing a more robust and reactionary defense mechanism.
- Machine learning models like random forest and neural networks are pivotal in extracting and analyzing features for phishing detection.
- Supporting a broad range of devices, including mobile phones, feature extraction ensures comprehensive security coverage.
- Meticulous evaluation of data features leads to an enhanced ability in preventing phishing attacks.
Adaptability to Evolving Threats
Threat intelligence plays a vital role in empowering machine learning systems to prevent phishing attacks effectively. By assimilating new patterns and strategies used by cybercriminals, machine learning algorithms stay ahead of the curve, always adjusting to counteract the latest phishing tactics.
In terms of email security, the adaptability of machine learning algorithms is paramount for phishing prevention. These systems swiftly integrate threat intelligence to recalibrate their parameters, ensuring continuous protection and the ability to prevent phishing attacks even as threats evolve.
Turning the tide in cybersecurity, machine learning not only identifies phishing attempts, it also paves the way for prevention. Let’s take a closer look at how ML-based strategies proactively guard against these nefarious schemes.
ML-Based Methods for Phishing Attack Prevention
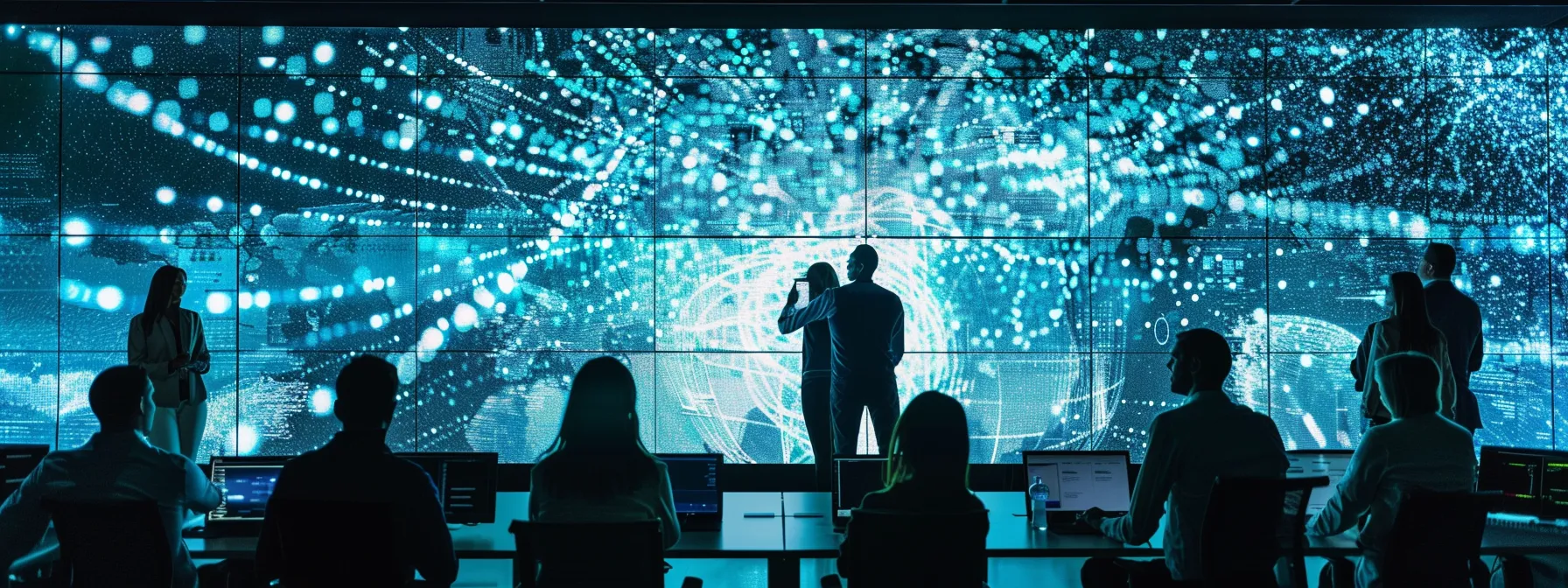
In the sphere of cybersecurity, the proliferation of phishing attacks necessitates innovative defense mechanisms. Machine Learning (ML) surfaces as an invaluable tool, enabling systems to analyze and comprehend multifaceted data in ways that traditional methods cannot. Social Graph Analysis and User Communication Profiling work in tandem, dissecting the web of user interactions and communication patterns to detect anomalies. Behavioral Analysis further scrutinizes user actions for signs of compromise or deceit, making it a potent ally against targeted phishing campaigns. Content Analysis delves deep into the subtleties within documents and messages, identifying malicious intent, while URL Analysis examines links to unmask the clandestine infrastructure of phishing sites. These evolving ML techniques collectively develop robust profiles and insights that fortify defenses against the cunning of cyber assailants, showcasing ML’s ascendancy as an essential component in thwarting phishing ventures.
Social Graph Analysis
Social graph analysis harnesses the vast potential of machine learning to enhance marketing efforts by mapping out the complex web of social relationships that exist within an organization. This analytical process regards the social network as a labyrinth of nodes and edges, representing individuals and their connections, to reveal insights into user interactions that might signal phishing activities.
In the domain of information security, management teams leverage social graph analysis to track communication patterns and behavioral links between employees. By applying sophisticated data analysis, organizations can detect irregularities that often precede a phishing attack, thereby strengthening their defensive posture against potential breaches:
NSCoder>
- Data analysis identifies atypical network patterns that suggest phishing attempts.
- Marketing teams can utilize insights from social graphs for targeted communications while remaining alert to phishing risks.
- Experience in interpreting social connections enhances information security strategies.
User Communication Profiling
User Communication Profiling employs sophisticated machine learning models, such as the naive bayes classifier, to dissect the intricacies of user behavior across various communication platforms. By analyzing patterns of credential input across emails and SMS exchanges, this form of profiling can detect anomalies indicative of phishing attempts that heuristic methods might overlook.
The method transcends the boundaries of heuristic analysis, adopting principles akin to physics, which seek to understand the governing laws behind observed phenomena. In this context, the naive bayes classifier systematically categorizes communication behaviors, distinguishing legitimate interactions from those manipulated by attackers to acquire credentials fraudulently.
Behavioral Analysis
Supervised learning, a subset of machine learning, fortifies the analysis of user behaviors, particularly within the context of web browser use. By employing this method, systems can effectively learn from past incidents of fraud, refining their capabilities through feedback to predict and preempt future phishing attempts.
Predictive analytics, bolstered by supervised learning techniques, transforms the fight against phishing into a proactive operation. This approach scrutinizes patterns of online engagement and anticipates fraudulent activities, allowing security mechanisms to intervene promptly before users fall prey to deceitful schemes.
Content Analysis
In the ongoing effort to bolster network security, Content Analysis emerges as a critical weapon, utilizing robust databases filled with phishing indicators. It cross-references each incident, building upon knowledge to discern legitimate content from phishing threats, thereby enhancing organizational defenses.
Content Analysis also capitalizes on DMARC (Domain-based Message Authentication, Reporting & Conformance) policies, using them as a reference point for verifying the authenticity of the communication. By aligning email checking processes with established DMARC protocols, systems enhance detection rates of phishing activities and secure email gateways effectively.
Anti-Phishing Method | Technology Utilized | Security Focus | Impact |
---|---|---|---|
Content Analysis | DMARC, Databases | Email Verification | Improves detection of phishing via verification against known threats |
User Education | Knowledge Transfer | Behavioral Awareness | Reduces susceptibility to phishing by informing users on latest tactics |
Machine Learning Detection | Predictive Algorithms | Network Security | Proactively identifies and responds to emerging phishing patterns |
Social Graph Analysis | Crossref of Social Data | Internal Communication | Detects anomalies in communication patterns within an organization |
URL Analysis
URL Analysis, empowered by a neural network, serves as a critical component in modern anti-phishing measures, meticulously evaluating the authenticity of web addresses. By scrutinizing the full spectrum of a URL‘s characteristics, from domain name to embedded parameters, neural network algorithms detect nuances and inconsistencies indicative of phishing websites. This method is particularly effective in shielding brands from the damage inflicted by phishing attacks that mimic legitimate sites.
Within the virtual community, URL Analysis extends its vigilance to the domain of social media where phishing attacks frequently masquerade as spear phishing campaigns. Leveraging machine learning, these analytical tools quickly parse URLs shared across social networks, identifying malicious links that exploit the trust of unaware users and aim to compromise personal or corporate accounts.
- Neural network algorithms are deployed for in-depth assessment of URLs to unmask phishing endeavors.
- URL Analysis targets the social media sphere to combat spear phishing attempts against individual users and established brands.
Frequently Asked Questions
What are the risks associated with phishing attacks?
Phishing attacks carry the risk of personal data breaches and financial theft. Victims can also suffer significant reputational damage if sensitive information is exploited.
Can machine learning significantly improve phishing detection?
Machine learning can markedly improve phishing detection by enabling systems to learn from vast datasets and identify threats that conventional static methods might miss. This technology adapts and evolves, offering a robust defense against an array of sophisticated phishing techniques.
How does machine fast-track the identification of phishing content?
Machines utilize sophisticated algorithms and machine learning techniques to quickly analyze and identify potential phishing threats. They can scan vast amounts of data, recognizing patterns and markers indicative of phishing content, thus expediting the detection process.
What techniques does machine learning use to prevent phishing?
Machine learning thwarts phishing by employing algorithms to detect suspicious patterns and validate emails against known threats. Additionally, these systems can analyze URLs and attachments within emails for potential risks, constantly improving through training with new phishing examples.
Why is machine learning more effective than traditional methods in combating phishing?
Machine learning outperforms traditional methods in combating phishing primarily because of its capability to analyze vast datasets quickly and identify patterns that signify malicious activity. While traditional methods often rely on predefined rules, machine learning continuously evolves, improving its predictive accuracy in real-time as it processes new data.
Conclusion
Machine learning fortifies cybersecurity by detecting nuanced phishing patterns and adapting in real-time to emerging threats. It surpasses conventional security methods through predictive analysis and intricate pattern recognition, delivering precise and proactive defenses. By integrating machine learning, organizations ensure a dynamic response to the constantly evolving tactics of cybercriminals, maintaining a robust security posture. Ultimately, empowering anti-phishing measures with machine learning is pivotal for safeguarding digital assets against sophisticated and clandestine cyber attacks.